IIoT technologies have enabled software platforms that are more powerful than ever, but fundamental engineering principles are still at the heart of next-generation digital transformation
It is clear that the emergence of industrial internet of things (IIoT) technologies has greatly expanded the capabilities of industrial software tools. Although IIoT's recent proliferation in the chemical process industries (CPI) may seem to be primarily driven by advances in software and other digital tools, such as artificial intelligence (AI) and cloud computing, these elements have built upon the types of solutions – as well as fundamental engineering principles – that have been deployed for several decades.
Software's digitalization journey
"The industry has been practicing digitalization for a long time, and the recent changes have made these applications more powerful, agile, portable, intelligent and autonomous. Evolution in the CPI is gradual; it is incremental due to the intrinsic liability associated with the operation of an industrial plant," says Sergio Fernandes, chemical market leader, Yokogawa Corp. of America (Sugar Land, Tex.; www.yokogawa.com/us). Nonetheless, CPI companies have successfully deployed digital technologies at a large scale, catalyzed in part by the shift from software being run on a user's laptop to the reality that high-performance applications and tools can now be accessed from almost literally anywhere. "Not only has cloud computing enabled a reduction in capital expenditure budgets, it has also facilitated the availability of process models, whether steady-state or dynamic, regardless of the end user's location," explains Fernandes. However, he cautions against simply assuming that a digital plant model, no matter how advanced, will be fully accurate in perpetuity. "Industrial processes are like living entities – they change with time. Any mathematical representation, such as a digital twin, needs to adapt and needs to be updated by some mechanism. Otherwise, it will eventually fall into disuse. Assets require attention; they demand budgets to maintain their sustainability," he adds. Envisioning a future with increasingly autonomous operations, the critical need for safety and sustainability means that a smart balance must be struck when deploying human resources alongside digital assets. "Risky field operations, repetitive actions, mundane activities, unnecessary trips to collect data in the field and inspection in hazardous areas can be intelligently addressed by current and upcoming technologies," says Fernandes, framing cutting-edge digital technologies as critical components that will enable an architecture that fosters more innovation from humans. "That means continuous improvement of operations, the anticipation of the next disruption and the optimization of the whole value chain."
Simply developing a software model that mimics a process or asset is not enough to truly capitalize on the promise of digitalization, reiterates Rajesh Ramachandran, chief digital officer of ABB Industrial Automation (Zurich, Switzerland; www.abb.com). "What's trending now is the industrial AI twin of a digital plant. This looks at how to predict and optimize a set of outcomes for a specific process scenario, giving the opportunity to fine-tune different parameters," he explains. Ramachandran emphasizes that pure AI cannot be applied "as-is" into an industrial context – corresponding domain expertise is essential to capture the complexities of CPI operations, such as the quality specifications for end products or the varying presence of feedstock impurities. ABB has combined data-driven AI with domain expertise built deep into the digital twin, ultimately building a cognitive model through its ABB Ability Genix software platform. "Genix builds something we call a cognitive model that expands upon advanced models with domain knowledge from different systems, such as maintenance, instruments and laboratories. What that means is that it helps to make more accurate predictions for optimization," adds Ramachandran. Citing industry studies that showed on average, a plant may only use around 27% of its produced data, while engineers may spend as much as 80% of their time aggregating data, he predicts that cutting-edge software platforms will help to alleviate these imbalances. "We are investing in fundamentally addressing areas of need to unlock the value in unused data and apply industrial AI at scale to get the maximum productivity and operational gains, while also simplifying the efforts toward data integration," he says.
Intelligent platforms
There is no doubt that industrial software platforms have gotten considerably more powerful in recent years, as more companies are embracing use cases for industrial AI and machine learning (ML). "In the CPI, these types of technologies are being integrated everywhere from asset monitoring to AI-powered drones than can inspect flare stacks," explains Michael Tworzydlo, product manager for analytics and machine learning at Emerson (St. Louis, Mo.; www.emerson.com). However, Tworzydlo cautions against overhyping the value of these solutions without acknowledging the importance of foundational engineering principles. "As chemical engineers, the fundamentals of analytics are the best starting place, and that begins with principles-driven analytics, based on physical laws, such as how a heat exchanger works. Then, organizations can evolve to data-driven approaches that leverage AI or ML for more complex processes or plant-wide issues," he adds.
"AI offers powerful capabilities to the CPI, but some companies struggle to effectively apply it to manufacturing challenges," explains Paige Morse, chemicals industry director at Aspen Technology, Inc. (Bedford, Mass.; www.aspentech.com). dc
As a response, AspenTech has begun embedding AI into its software platforms, which makes it more accessible to a broader mix of users. The combination of first-principles engineering with AI and domain expertise, Morse notes, can help users to better find solutions for the complex problems that must be navigated in the CPI. AspenTech's Hybrid Models approach can not only aid in process optimization, but can also empower engineers to create custom soft sensors, design new equipment and integrate asset-wide processes. "Engineers can now build enriched models faster using ML to leverage simulation or plant data, adding domain expertise, engineering principles and design constraints, without requiring deep process or AI expertise," says Morse. As many CPI companies are facing legitimate skills gaps, technologies that empower workers to tackle tasks that were previously reserved for experts or specialists are especially vital.
In addition to overcoming workforce gaps, sustainability initiatives are another area where CPI companies are increasingly focusing their efforts. "Cost savings have driven many digitalization efforts, but companies are increasingly focused on waste and emissions from production units, as well as efficiency and reliability enhancements," says Morse. "Process simulations aid the development of new products to address the technical challenges of the circular economy, such as molecular recycling and new plastic design, and this activity moves even faster when aided by AI," she adds.
Such predictive capabilities are increasingly valuable in meeting specific sustainability goals, such as reducing air pollution with predictive emissions-monitoring systems (PEMS), a functionality of Emerson's Plantweb Optics Analytics platform (Figure 1), which deploys ML and AI with digital twins and distributed control systems. "As part of Plantweb Optics Analytics, we can deploy PEMS that monitor and estimate emissions using models and ML to dynamically optimize production. With PEMS, we can build models based on process variables that we are already capturing and use those to estimate, and ultimately reduce, emissions," says Tworzydlo.
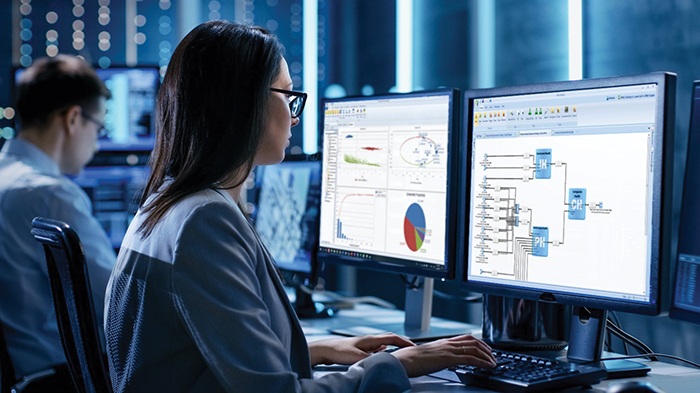
FIGURE 1. Along with having a view of the process in a digital twin, an analytical software platform can provide automated root-cause analysis on any process issues or deviations, including recommended actions
Software's promise to help deliver on sustainability strategies stretches beyond emissions reduction. "The rise of sustainable products and technologies to reuse or recover waste have been growth areas for the process simulation industry, creating new problems and new opportunities. Recent growth areas include process simulations of marijuana derivatives, such as CBD, as well as improved control to reduce venting of renewables. More established growth areas for the industry have included biofuels, methane recovery, CO2 recovery and solvent selection," says David Hill, manager of technical support at Chemstations Inc. (Houston, Tex.; www.chemstations.com).
Hill believes that the promise of process simulators will be further enhanced through product alliances with complementary tools in the CPI. " Engineers who don't use process simulators often have tools that can be improved by connecting to a process simulator. There are many opportunities in safety, process control, and energy areas to combine industry-specific tools with the first-principle calculations of a process simulator," explains Hill. The driving factors for this transition, according to Hill, will include opportunities for greenhouse-gas reduction, energy efficiency, optimization based on thermodynamic models, improved safety and advanced process control using rigorous simulations.
Extending reality
In addition to AI and ML, augmented reality (AR) and virtual reality (VR) software platforms are also on the rise in industrial plants – and owing to the increased need for remote work during the pandemic, these sorts of technologies are no longer seen as "luxury" items and are more useful than ever. "Due to the pandemic, fewer people are in plants, and plants are embracing new technologies. AR allows digital information to be overlaid onto the real world, and this helps better equip workers to complete tasks accurately and with fewer interruptions," says Emerson's Tworzydlo. As for the future of AI, ML and AR in industrial software, the use cases will surely continue to expand. "There is still a lot of untapped potential. Eventually, we will start moving into autonomous operations for certain processes, where those processes increasingly become self-diagnosing and self-healing," Tworzydlo predicts.
Aveva Group plc (Cambridge, U.K.; www.aveva.com) has bundled AR and VR concepts into extended reality (XR) platforms, with one particularly pertinent application being personnel training (Figure 2). "XR immersive training systems allow companies to capture and retain operational knowledge during the replacement of retiring experienced operators, which is critical for plant safety and performance. This behavioral training can be applied not only for front-line operators, but also for engineers, technicians and emergency personnel," explains Ravi Gopinath, chief cloud officer and chief product officer at Aveva.
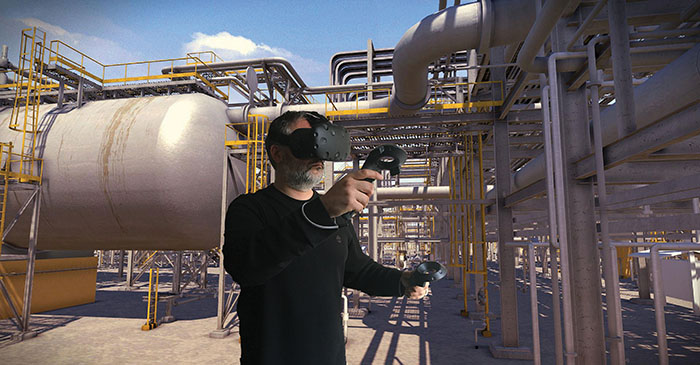
FIGURE 2. Building upon existing training technologies by using extended reality and the cloud, along with digital-twin capabilities, promises significant safety benefits and cost savings
In one instance, an operator-training scheme developed by Aveva and Shell (The Hague, the Netherlands; www.shell.com) focused on behavioral training to increase safety competencies. "With this behavioral approach, the operator can be trained and assessed on how he or she will behave when faced with unexpected or infrequent situations in the plant," says Gopinath. In another project, Aveva and Adnoc (Abu Dhabi; www.adnoc.ae) created a real-time data-visualization center that brings together over 120 dashboards and 200,000 data points on a massive interactive screen.
Training is just one part of XR's potential. AR tablet-based applications are already being used to support field workers. Connecting the VR model in the tablet to real-time information and guided procedures with AR enables better work execution, preventing costly failures and reducing downtime. Looking toward the future, Aveva believes that XR software will significantly improve facility design and capital project engineering by enabling the automatic import of conventional 3D plant models, which are used during the design phase, into an immersive environment. "This conversion into VR will allow the ergonomic design to be reviewed and improved before any equipment is even purchased. The virtual plant can already exist entirely in the cloud, allowing collaboration between engineers at different offices or even on different continents. With this approach, the project can access diverse expertise without any travel and the design review is executed faster, with less cost and risk," emphasizes Gopinath.
Looking outside the box
As software platforms' data-capture and analysis capabilities have progressed, powerful analytics tools have become self-service, scalable decision-making tools for chemical engineers, into which they can build their own functionalities to meet specific process needs. With such democratized tools, engineers can leverage data from disparate sources – for instance, laboratory information like batch quality can be linked to process data alongside maintenance data, says Edwin van Dijk, vice president of marketing at TrendMiner N.V. (Hasselt, Belgium; www.trendminer.com). "The goal of democratizing analytics is to enable everybody in operations, from control room to boardroom, to use actionable insights for making data-driven decisions. This goes beyond traditional dashboarding tools by allowing the users to create their own dashboards based on fingerprints, monitors and context views," adds Van Dijk. Through pattern recognition, engineers can investigate operational performance and use good operational behavior for process monitoring. Furthermore, they can create their own "soft" sensors to monitor what physical sensors cannot measure, such as product-quality specifications.
A data-analytics success story reported by TrendMiner involved a chemical plant that was experiencing "sticky" valves, which were creating a delay between the valve's output changing and the actual process responding. The plant wanted to accurately identify when a valve was beginning to stick, so they needed to monitor for any deviations from the valve's expected behavior and then find the parameters that distinguished between periods of "good" and "bad" valve behavior. These parameters were translated into alerts for out-of-phase behavior that would not only notify personnel about the situation, but also suggest possible corrective actions (Figure 3). "By using the self-service analytics solution, the process experts were able to use high-speed trend analysis to search and validate production issues, using embedded AI and ML capabilities," explains van Dijk.
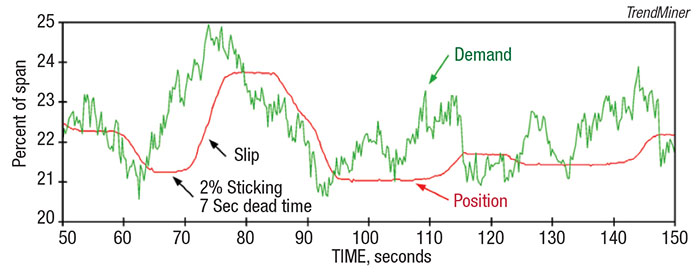
FIGURE 3. Advanced analytics techniques can help engineers with an in-application recommender engine, suggesting potential root causes for process issues such as
valve stickiness
Even with the vast selection of software tools and mobile apps available, some users still require highly customized solutions to meet their business needs. This is where in-house programming can come in handy. JourneyApps (Denver, Colo.; www.journeyapps.com) offers a high-productivity app-development platform, with which users can write their own code, enabling more complex apps than no-code app-building tools, which are aimed at non-programmers and are limited by their simplicity. "This means that advanced business logic, engineering calculations and highly customized integrations can be implemented in a matter of days without much of the traditional overhead associated with software development," explains Conrad Hofmeyr, CEO of JourneyApps. He points out that most chemical engineers have some basic coding or scripting experience through tools like Microsoft Excel Macros or Matlab, so they can quickly pick up the necessary programming skills to use JourneyApps to build sophisticated apps that automate and streamline key business functions.
For instance, Hofmeyr cites an example where a CPI company built a dedicated app for standard operating procedures (SOPs) that enabled them to shift from manual spreadsheet-based SOPs to a centrally controlled app with full audit trails. "The customizability that JourneyApps provides meant that a single, global app could be customized to local requirements and system integrations," he adds. In another example, an oilfield-chemicals manufacturer developed its own app to run key calculations used in its daily field-reporting processes and generate reporting documents, all while users are offline at disconnected sites.
Looking toward end uses
Advanced software and modeling tools are also enabling the creation of safer, more effective end products in many industry sectors, from automotive parts to pharmaceuticals. One example is BASF SE's (Ludwigshafen, Germany) Ultrasim computer-aided engineering (CAE) tool for simulating materials behavior (Figure 4), which was recently updated to model an extended range of thermoplastic elastomer materials over the entire process chain, from initial processing to end-use products. "Shrinking development cycles and aggressive timelines put an increased amount of stress on engineers to get product performance right the first time. Having predictive accuracy offers tremendous advantages," says Marios Lambi, team leader for BASF Simulation Engineering CAE, North America. Ultrasim enables the simulation of both initial load and cyclic loads on a component, which proves to be particularly essential for automotive parts made of elastomeric materials. "From creep loads to crash simulations and thermal loads and vibrational behavior, coupled with processing simulations, which describe process-induced material properties, and numerical optimization tools, which allow fast geometrical variations, Ultrasim builds the basis for designing better parts," emphasizes Andreas Wüst, team leader for BASF Dynamic Structural Analysis, Europe.
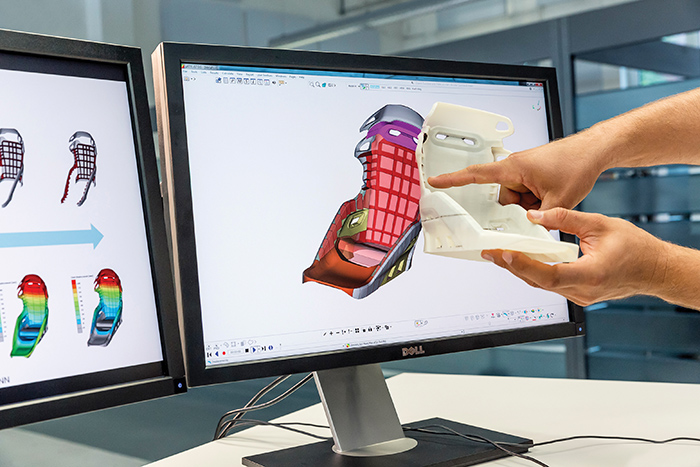
FIGURE 4. Ultrasim involves models that incorporate anisotropic (directionally dependent) fiber orientation in a mechanical simulation of a technical part, along with materials models that look at the dependency of the material behavior to the speed of deformation
The foundation for ensuring accurate materials simulation is the underlying data. "The material-characterization process generates the necessary data that are essential in the accuracy in predicting real part behavior. The theoretical material models developed for this purpose are using the information from testing to calibrate and therefore ensure the behavior represents real manufacturing conditions and not an arbitrary case that is far from reality," Lambi points out. "There are complicated assembly examples, such as automotive seating, which are subjected to crash testing that utilize Ultrasim's predictive accuracy to build parts that pass validation testing. This dramatically reduces the development cycle and minimizes, if not eliminates, design changes," he adds. Ultrasim also provides capabilities for the growing market share of 3D-printed parts, which can now be investigated using the tool, further accelerating the cycle time from design to prototype to end product.
For the high-precision processes in research and development and quality-analysis laboratories for biopharmaceutical ingredients and other high-value products, software tools can serve several purposes, including the facilitation of an organization's business continuity plan (BCP). "When looking at the laboratory, efficient software can mitigate or reduce the number of risks, streamline testing of events, have automated procedures to restore the system once an event is over and even keep the system running during an event, all simplifying the BCP," says Barbara van Cann, product marketing manager for chromatography software in the Enterprise Chromeleon Data System organization at Thermo Fisher Scientific (Waltham, Mass.; www.thermofisher.com). Moreover, laboratories can further streamline their BCP by selecting integrated software that encompasses the chromatography data system (CDS), the laboratory information-management system (LIMS) and the laboratory execution system (LES). "Both LIMS and CDS software should provide tools to monitor instrument qualification, calibration and maintenance, even down to individual component parts," explains Van Cann. The CDS software should also help users to deal with analytics irregularities, as well as have built-in network failure protection to ensure continued operations, without manual interaction, in the event of network outages. To avoid outages due to cybersecurity attacks, Van Cann advises that laboratories run CDS and other software on a domain separated from main office systems to avoid potential cyber-threats originating from emails. Finally, as with any automated software platform, the human element must be considered. "Human error can be reduced by controlling what users can and cannot do, and can and cannot access. In addition, there should be tools to automate as many actions as possible. Less user interaction equals fewer errors," she adds.