The terms "cloud" and "edge" are used frequently by tech-savvy power industry professionals, but what do they mean and how are they changing plant operations? To get an insider's perspective, POWER posed the question to Akshay Patwal, strategic business manager with Siemens Energy. Patwal leads the development and commercialization of digital business transformation projects, using big data platforms and analytics, to create data-driven services. He has been pioneering the building of digitalization and big data-based framework by developing and implementing company-wide platforms, systems, and business processes to maximize the value of enterprise data, driving performance improvement, cost reduction, and revenue generation. He is an expert and thought-leader on data management, data analytics, and visualization technologies in the energy industry, so he obviously has a wealth of knowledge on the subject.
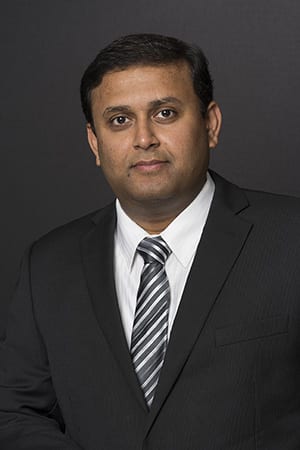
Akshay Patwal is strategic business manager with Siemens Energy. Courtesy: Siemens
POWER: What do cloud and edge mean, and why is that technology important?
Patwal: Due to the exponential increase in the number of devices in the IoT [internet of things] network, there has been a significant increase in the data storage and processing power of these devices. In cloud computing architecture, all the data generated in the IoT network is moved onto a centralized cloud location and processed for specific goals. This creates a situation where all the devices need to have access to data or applications in the cloud. It offers a secure way of handling data with remote access to it. One of the biggest advantages of the cloud is it ensures that only authorized users can access the information and applications needed.
However, this centralized setup becomes a constraint in terms of processing data collected at the edge of the network quickly with low latency. But cloud has tremendous processing power and capacity, and is highly scalable, which makes it an important entity in data management and processing.
But, this centralized way of processing data has led to a few problems in the scenario where there is a huge amount of data being generated at the edge of the network, and recently those devices have gained excellent processing power. Firstly, the time taken for data communication–from edge device to centralized cloud and back–is significant. It also puts a lot of strain on the network bandwidth and can slow down the network tremendously. Edge computing offers a solution to this issue by housing the key device data at the edge itself and processing it in real time to solve the problem locally. As a result, less bandwidth and processing are needed without straining the network. It offers a versatile approach to network infrastructure by using the processing power available through the combination of modern IoT devices and edge datacenters.
But one of the major limitations of edge devices is that they only accumulate locally collected data, making it difficult for them to utilize any kind of "big data" analytics. Cloud computing solves this by allowing large-scale data analytics, which is beyond the edge and allows artificial intelligence (AI) and machine learning (ML) to be more viable.
POWER: How can plant technicians and managers decide when and where to use these tools?
Patwal: The best way to use the best of both the worlds is to incorporate edge computing with cloud computing, by combining the data-acquiring potential of the edge with the storage and processing potential of the cloud. The big question would be when to use edge over cloud. Some of the following points could help answer this question.
Connectivity. Connectivity is erratic in highly distributed infrastructure environments and leads to severe strain on the network bandwidth for data transfer. Cloud is heavily reliant on computing power and bandwidth, and legacy systems aren't designed for this type of data connectivity. Therefore, they will not be able to support the IIoT based on use cases needed by the cloud.
Immediacy. The speed of decision-making is key in the implementation of edge computing, as in some cases instantaneous decisions need to be taken to prevent catastrophic failures.
Volume. As the data volume grows, generally its pattern remains the same, but for a few instances. When it is sent to the cloud, it is filtered and edge provides that key monitoring of this process. In addition, because ML cannot operate off raw streaming data, edge can prepare the data for it.
Cost. In some cases, the cost of building infrastructure to support the cloud is expensive, as it needs high rating devices, stronger connectivity, bigger storage, and more. Edge tones down this requirement, being very economical for the same use cases.
Privacy. Very often, regulations and customer concerns prohibit the data transfer out of their domain, but they still expect to obtain the analytical service needed. Edge offers an excellent solution where it houses the data and processes it locally.
Security. Security vulnerabilities are minimized by limiting physical and cyber access to the required assets. It prevents wrong operation and unauthorized use while providing intelligence to required resources.
POWER: Will edge analytics eventually replace the cloud?
Patwal: I think edge will complement cloud by driving near-real-time data management and analytics close to the data source. Edge will reduce cost using a distributed computing architecture to process critical analysis, reducing cloud dependency. For combining edge-to-cloud architecture, the real-time analytics needs to run at different levels, such as sensor, machine, gateway, or controller in the on-premise or cloud environment. The introduction of smart sensors at the edge is resulting in it making smart decisions, and later synchronizing with cloud. As a result, a well-defined edge-to-cloud architecture–as per domain and data–would accelerate cloud computing.
On the question of which one should be used over the other, I believe they cannot be looked upon as separate computing systems, instead, they need to complement each other. The main areas of focus in a power plant are:
- Increasing Availability. The optimization of power plant equipment generally takes place as a pre-planned scenario based on asset history and operations. I believe cloud computing should be used here, but edge can analyze the equipment behavior locally along with other data and complement the cloud analysis. This will further strengthen the cloud analyses to regulate maintenance activities and optimize condition-based maintenance with the balancing of operations and maintenance activities with reliability and inventory management.
- Performance Improvement. The same approach as above needs to be implemented for performance improvement, as cloud can look at historic performance data combined with relevant factors, and edge looks at the real-time performance patterns to guide the cloud analysis. This provides a more in-depth view of the behavior and helps continuous degradation recovery.
- Remote Fault Detection and Diagnostics. This is one area where I believe edge can gain priority over cloud by detecting unexpected faults and performing diagnostics. But the learnings and results of the task can be shared with the cloud computing when its performing life and performance calculations of the asset.
- Remote Field Support. Again, edge gains preference here as it will guide the monitoring and diagnostics of power plant assets to have early detection of the anomalies and impending damage to them. It will also deploy the right workforce and inventory required for the recovery. But, as in the previous case, the results are incorporated in the lifing and performance calculations in the cloud.
- Risk and Compliance. Cybersecurity is definitely an edge analysis, as it has a faster response to cyberattacks, and often utilities are skeptical in sharing their network with third parties. But, one area where cloud can come into the picture is that these cyber scenarios can be input into cloud analysis to design stronger and customized cybersecurity packages for the future.
- Fleet Management. Cloud computing gains ascendancy here as a centralized cloud has the overall view of the fleet. It can perform monitoring and diagnostics for full transparency across machine, plant, and fleet level, and provide asset diagnostics, performance monitoring, and operations optimization to create new avenues for utilities to manage and market their assets. The role of edge is to provide local machine level or plant level monitoring and diagnostics to the larger analysis.
–Aaron Larson is POWER's executive editor (@AaronL_Power, @POWERmagazine).