The term "Big Data" is used frequently by tech-savvy power industry professionals, but what does it really mean. To get an insider's perspective, POWER posed the question to Akshay Patwal, strategic business manager with Siemens Energy. Patwal leads the development and commercialization of digital business transformation projects, using big data platforms and analytics to create data-driven services, so he has a wealth of knowledge on the subject.
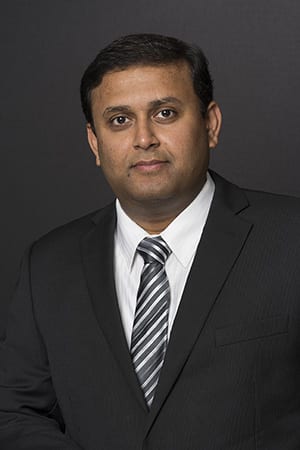
Akshay Patwal is strategic business manager with Siemens Energy. Courtesy: Siemens
POWER: What does Big Data mean to you?
Patwal: Big data in power generation refers to the large amounts of data being obtained from various sources in the power plant. The magnitude we are looking at here would be in terabytes of data or even more. The big data has the following characteristics:
- A huge volume of data is expected and so would be the storage space needed for it. Therefore, the hardware and software infrastructure need to be continuously upgraded to meet the volume increase.
- The rate at which the data is generated ranges from sub-second to minutes, and real-time data acquisition and management approaches need to be adopted.
- The data generated is in different formats, including text, images, video, spreadsheets, and databases.
Broadly, there are two types of data:
- Asset Operating Data. This data is obtained from the various sensors embedded in the asset to measure various operational metrics, such as temperature, pressure, efficiency, etc., of the asset or a group of assets. This data is structured in a way that it can be laid out in a spreadsheet along with a time stamp when the data point gets generated. It provides insight into the operating condition of the asset at regular intervals, and the interval can range from sub-seconds to minutes. The higher the data resolution, the more insight can be obtained from it.
- System Data. The other key data is called system data, which provides information on the condition of the asset, its maintenance records, installation records, repair records, part exchange details, photos or videos of the asset, etc. This information is unstructured in nature and cannot be laid out in a spreadsheet as in the case of operating data. Some of these records could be free text and advanced analytical methods such as natural language processing needs to be implemented for its analysis. One of the challenges is to convert this unstructured data to some sort of a structure for analysis.
There are other types of data, such as weather conditions, plant reliability data, asset outage data, etc., which play an important role in understanding the behavior of the asset in terms of its performance and maintainability. There is a common term used in the power generation world called "RAM," which stands for reliability, availability, and maintainability. All data analytics are generally targeted to optimize these three key metrics.
POWER: How does Big Data benefit power companies?
Patwal: The true value of the data is possible only when the data with different volume, velocity, and variety is integrated into a dataset, which can be analyzed, and relevant analytics are performed on it to understand the whole picture about the asset behavior, customer business models, and market dynamics.
Big data–even though very useful in understanding the asset behavior, customer business models, and market dynamics–poses a lot of challenges. These include:
- Data Growth. One of the most basic challenges for the power generation industry is storing and analyzing the terabytes of data. Due to the sub-second resolution of data generation, it is predicted that the data will double every two years. To add to the complication, a good chunk of that data will be unstructured and will need special processing to be converted to a usable format. The data management and data analytics platforms need to be envisioned to tackle this data challenge and need to be combined with the domain expertise to obtain usable insights.
- Data Acquisition and Storage. Acquisition of data from different sources requires strong connectivity, excellent ETL (extraction, transformation, and loading) routines, and relational storage databases. Connectivity is key in ensuring that the data is transferred from the source to the data landing zone with limited disruptions and low latency. The ETL process should be able to check for data availability, transform unusable data formats to a usable one, and ensure that the data gets loaded accordingly. The databases used need to be relational in nature, that is, they must store and provide access to data points that are related to each other and represented in tables.
- Data Validation. Validating data obtained from various sources is an important aspect of big data management. Data governance is key as the data should be checked for correctness, credibility, reliability, and content to ensure that it can be used for analysis. All the analyses in the industry process the relevant data, but to have accurate and meaningful insights, the data needs to satisfy the above-mentioned criteria. The data needs to be correct in terms of values and content. The values provided should be credible and reliable so that users can believe them. More importantly, there should be low disruptions in the data streams to minimize gaps in data. For a strong and simplified data governance, there needs to be a combination of policy and technology changes including an effective data management framework.
- Data Security. Data security has gained significance due to the value the data brings to an organization. Prevention of data hacks, cyberattacks, malware, and viruses is quite high on the priority of companies due to the fact that incorrect analysis or exposure of data to wrong parties can cause irreparable damage to the organization. Some of the popular data security approaches adopted recently have been identity and access control, data encryption, and data segregation.
- Data Integration. As mentioned earlier, the volume, variety, and velocity of data poses a challenge in integrating them into a usable format to be analyzed. Combining structured and unstructured data with different resolutions, formats, and units is highly complicated. The true value of data can only be accessed when the data engineer is able to analyze this combined dataset.
- Relevant Insight Generation. The insights obtained by analyzing big data needs to be relevant and in a timely manner. Just analyzing data to obtain the obvious insights doesn't help any organization. There should be targeted analysis of the dataset intended toward cost savings, revenue increase, output increase, improved reliability, optimized maintenance, new business model creation, etc. Companies need to extract insights and devise prescriptive recommendations to be more competitive in the market with a better understanding of the industry and customer behavior.
- Big Data Talent Management. Dealing with various aspects of data management and analysis needs special talents and skillsets. Due to the sudden prioritization of data as an asset to organizations, there has been an increase in the demand for the talent needed in this regard. There has been a race among companies to recruit and retain key big data experts and big salaries are being demanded. Companies are forced to increase their budgets, recruitment efforts, and training opportunities to develop the talent needed. Acquisition of big data startups and tools is also on the rise.
- Organizational Culture. In addition to the technological and logistical challenges, the other key challenge has been the adoption of this new approach by the organizational culture. Due to varied reasons, including insufficient organizational alignment, lack of understanding among the various levels of organizations, and resistance to adoption by the users who are comfortable with the traditional ways, digital approaches are not fully integrated in many organizations. Investment in new strong leaders, who understand the data world and can challenge the business, is the need of the hour. A new role of a chief digital officer–along with enterprise-wide executives, directors, and managers who can overcome big data challenges–has to be laid out if companies want to be competitive in the ever-increasing data-driven economy.
–Aaron Larson is POWER's executive editor (@AaronL_Power, @POWERmagazine).